.png)
The promise of generative AI (gen AI) has shifted the day-to-day focus of most CIOs. Responsible for adding business value, driving digital transformation, and managing change, gen AI adoption lands squarely in their court.
Employees are enthusiastically requesting new tools, point solutions, and training. Meanwhile, the market landscape and regulatory considerations make it hard to give easy approvals.
While every company will likely equip their teams with AI, returns on investment will be completely dependent on adoption. In this article we offer a maturity model for three stages of AI adoption, including strategies for company leaders to progress to the next stage.
The 3 Stages of AI Adoption
Stage One: Solutions Thinking
At this first stage of implementation, many CIOs are tasked to make bets and deploy budget toward an ROI that’s still hard to predict. Their thinking is solutions-focused; pick an option that solves a discrete need and has low-risk to workflow disruption. We typically see CIOs look at two solutions at this stage.
AI Add-Ons to Existing Vendors
An obvious option lies with existing vendors; many big solution providers, like ServiceNow or Salesforce, have released AI add-ons that promise additional returns against a specific business problem. At this stage, the decision seems simple: purchase these upgrades and realize AI gains without any security risks or disruption to existing workflows.
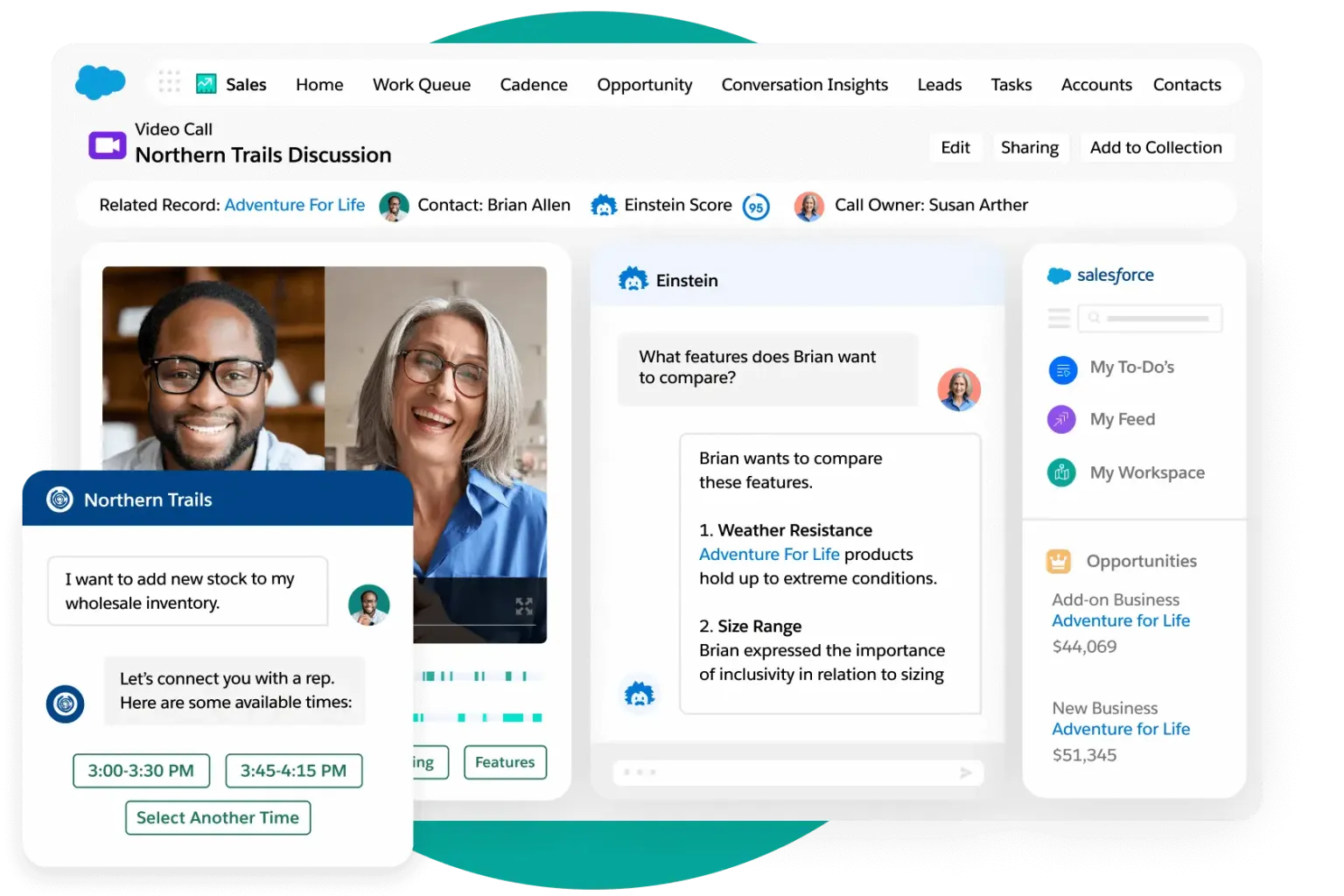
As more vendors release AI offerings, more teams request upgrades. Every department can imagine a transactional benefit from a new AI tool; a faster first draft, a better search, a more efficient research assistant. At this stage, employees ask for one-off tool subscriptions or in-app upgrades that are specific to the goals of their respective departments. Here, CIOs have to stagger their investments, and prioritize a fraction of the requested upgrades based on the highest expected ROI.
Internal AI Build-Outs
Most in-house AI investment is directed to data science and engineering teams. Technical employees are encouraged to use AI to improve existing models and projects. These in-house projects are long, complex, talent-intensive, and solution-specific. There’s an understanding that any high-value implementation of AI will be the result of these data science-led builds, and the company will see ROI once the solutions are deployed.
.png)
These projects are typically reactionary as business needs come up, and tailored to specific department requests. Data teams may implement gen AI through glue code and Python scripts to build a topic model for customer success teams, or a classification system for contract analysis. Technical experts lead the implementation, and non-technical teams see the end result. But handing off projects often comes with friction. Most solutions require business teams to re-task their data science organization with adjustments and iterations, and struggle to use the solutions beyond their pre-defined purpose.
This stage is talent and budget intensive. The cost of big vendor add-ons is significant, and complex in-house builds require expensive hiring. The expected ROI remains mostly unknown.

Stage Two: Siloed Implementation & Expanded Expectations
While expensive and talent-intensive, positive signals at Stage One encourage further exploration. Non-technical teams, having gained some experience with gen AI platforms and point solutions, are eager to use gen AI to augment more of their work. Technical teams also continue their exploration, looking to more flexible solutions like open source tech.
Non-Technical Adoption: AI Side-kicks
At this second stage, gen AI is understood as an employee’s personal side-kick. There’s a broad belief that the productivity gains from copilots and chat interfaces, like OpenAI’s ChatGPT, Microsoft’s Copilot, and Anthropic’s Claude, will lead to specific productivity gains that warrant investment. Point solutions, like Jasper.ai for marketing, Apollo.io for sales, and Perplexity AI for writing and research, are similarly useful in gaining efficiency for specific tasks across almost all areas of the organization.
.png)
These tools have proved their worth; many ‘power users’ have reported improvements in their motivation and enjoyment, creativity, and ability to focus on their most important tasks. In contrast to Stage One, non-technical users are interfacing with AI, learning how to prompt and give more context to these tools to achieve their desired result.
But conversation-style gen AI interfaces are model-specific; to get enterprise-grade licenses, your organization needs to lock in to one LLM provider. And since most of these tools are removed from business data, or are performing black-box operations on business data in a templated way, they can't fundamentally change how an employee's work gets done. Returns are table stakes.
Technical Adoption: Open Source Solutions
Technical teams at this stage have evolved from department-specific projects and into organization-wide models, often using open source solutions like Mistral and Llama 3 to give them the flexibility they need. While this is an accelerated version of Stage One, the work is still siloed from non-technical teams; they won’t interact with the output of this work directly. Still, organizations continue to deploy effort and budget into these projects in hopes of staying ahead of the curve.
AI Education & AI-Specific Hiring
This is the first stage where organizations start to deploy budget into both training their employees on AI usage and hiring for AI-specific talent. Some budget is allocated to improve employee productivity with the platforms and point solutions they have–namely through teaching employees about prompt engineering with training courses specific to the vertical tools that were purchased. The rest of the available budget is dedicated to hiring more AI-specific engineering talent to further the technical projects.
While this work remains talent intensive, more exploration is happening across the wider workforce, and competing for technical talent is less pressing. Some teams at this stage have found signs of significant cost savings and returns from increased employee productivity. Here the ROI is starting to show, but real returns are capped by the inherent limitations of copilots and chat interfaces that keep employees from embedding gen AI into more of their real business work.
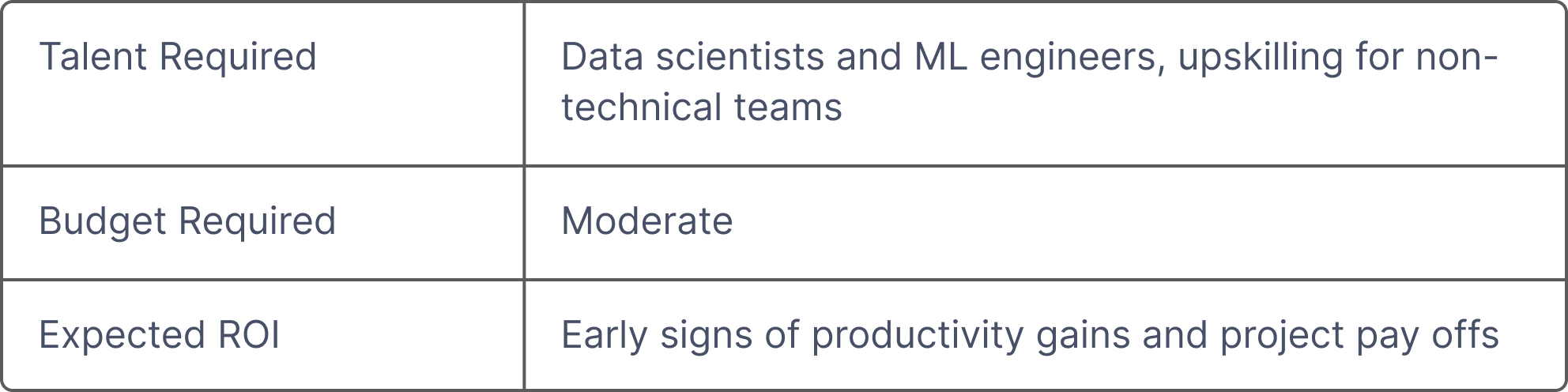
Stage Three: Integrated Use & Uncapped Upside
While we're still early in the 'final' stage of workforce-wide gen AI adoption, early adopters and market reports are showing the value it brings. The companies that have implemented multiple active applications of gen AI throughout the organization have 43% of their teams using the technology near-daily for multiple aspects of their work.
AI-Integrated Workflows
At this stage, gen AI has graduated from an employee’s assistant to a driver of their everyday work, empowering them to solve new problems and do more of what they do best. With access to large language models (LLMs), non-technical teams are working with more data than ever before, and complex analyses are newly accessible to domain experts–the people closest to that part of the business. They’re extracting key insights from their data in minutes instead of months, without the need of technical help.
One-off subscriptions and point solutions are being replaced by robust gen AI workspaces. Here, teams can leverage multiple models in an environment that's connected to their data sources. This reduces budget demands and compliance concerns by centralizing access to data and gen AI across the organization. In contrast to prior stages, CIOs and IT teams can breathe a sigh of relief!
Most notably, non-technical employees have moved from being consumers of AI to producers of AI. A user-friendly, safe, and data-connected interface allow them to:
- Combine multiple data sets and use prompts to analyze or transform them–without technical knowledge
- Create new classifications and workflows that reflect their domain expertise
- Collaborate across teams by sharing prompts and data to do more work and solve new problems
Investing in Cross-Functional Skills
Instead of focusing AI education on specific tools, training and up-skilling at this stage is focused on developing core critical thinking skills. Employees are equipped to break down a problem and embed gen AI into simple and complex tasks. As employees learn about both data engineering (how to curate their data) and prompt engineering (how to interact with it), they can leverage gen AI for a wide range of unique processes and needs. Resources that help employees leverage gen AI in tandem with basic data engineer practices are helping teams come to data-driven, validated conclusions without overburdening data and engineering teams.

Improved Technical AI Efficiency
With more access to data and models, technical teams are diving deeper into their AI projects. Many teams are building or fine-tuning proprietary models, building AI-powered products, or working on big picture solutions. Even the most technical work is accelerated with an integrated approach to gen AI, and as technical teams become more efficient in their work, the pressure to compete for additional technical talent in an expensive hiring market is drastically reduced.
In this stage, companies are generating talent; employees across domains are up-leveling the way they work in real time. Technical teams can focus on building the best AI foundations, knowing that non-technical teams will improve those models with their domain expertise. Here, companies are getting beyond the initial promises of productivity and efficiency, and starting to capture the returns of a value-creating workforce. To suggest an ROI would be to impose a false ceiling; these teams are limited only by the solutions they can imagine.
