.png)
Retrieval augmented generation (RAG) is an architecture for grounding large language models (LLMs) that’s seen fast adoption. Early applications have been particularly useful for customer-facing chatbots, improving response accuracy, reducing hallucinations, and helping teams deliver consistent, business-specific information to users at scale.
RAG systems are complex; development and maintenance requires constant testing and oversight. This article walks through a process of improving RAG performance, beginning with the most fundamental requisite to success: knowledge base maintenance.
What is RAG?
RAG is a technique through which a generative model is provided useful context from a curated external knowledge source. By adding information retrieval to text generation, a RAG system allows companies to add protocols, policies, and product information to the LLM’s pretrained knowledge to return safe and tailored answers to customers.
RAG is particularly useful in question and answer settings where the information is company- or product-specific, and unable to be extrapolated by the LLM.
.png)
How does RAG work?
A RAG system first identifies and isolates the question contained within a user’s input and queries the knowledge base, searching for relevant information. It identifies the relevant knowledge article, retrieves relevant chunks, parses them, and returns the answer. The LLM generates an output grounded in that answer, and the output is returned to the user; a generative response augmented by retrieved information.
RAG operates across three disparate areas of Natural Language Understanding (NLU): document ingestion, document retrieval, and generative response. Success depends on a series of technical steps: finding the closest matching knowledge article, parsing the right chunks, and creating logical answers with the optimal balance of grounding controls.
But there’s a simpler and more primary question that begins the process of RAG optimization: does the knowledge base contain the answers users are looking for?
What is a Knowledge Base?
A knowledge base is a data store of structured information–a centralized repository of up-to-date product information, services, policies, and procedures specific to a company. This is the source of truth for customers, employees, and the RAG system, allowing all three to provide and receive accurate, consistent information.
As in the case of an employee, the success of the RAG system is entirely dependent on the quality of the information it’s given. Knowledge isn’t static–it changes with new features, use cases, and user personas–and knowledge base completion is a continuous initiative.
Until now, companies have built and evolved their knowledge bases in isolation, guessing at what their customers need to know. But the advent of LLMs affords a more efficient and customer-centric process of understanding what the knowledge base covers, what users are searching for, and what gaps need to be addressed in what order.
Finding Knowledge Base Gaps with LLMs
LLMs are the perfect partner through which to test a knowledge base. With the right data environment, an LLM can work across all available knowledge articles to help teams understand what they cover.
For example, a ‘topic extraction’ prompt could delineate the themes contained within the articles, similar to subheadings and titles. While this is certainly faster than manually scanning, a list of topics doesn’t fully represent how the content would fare with real user questions.
If instead we ask the LLM to generate examples of questions the knowledge articles could answer, we’ll have an apples-to-apples comparison. In the above demonstration, we use the following prompt:

.png)
Running this prompt across every knowledge article at once gives us a long list of addressable questions that represent the coverage of the knowledge base as it stands. These examples can be grouped and labeled by topic. This is the new library against which we can backtest real user questions to see whether they would have been covered, or whether the RAG system would have been at risk of hallucination due to a lack of information.
Comparing User Queries to Knowledge Base Content
Within the same data environment, we can upload user conversations across customer support emails, user forums, and social media touch points to understand their questions in their own words. In the demo above, we’ve uploaded posts from a fictional user forum. We engineered the following prompt to isolate the users’ questions:
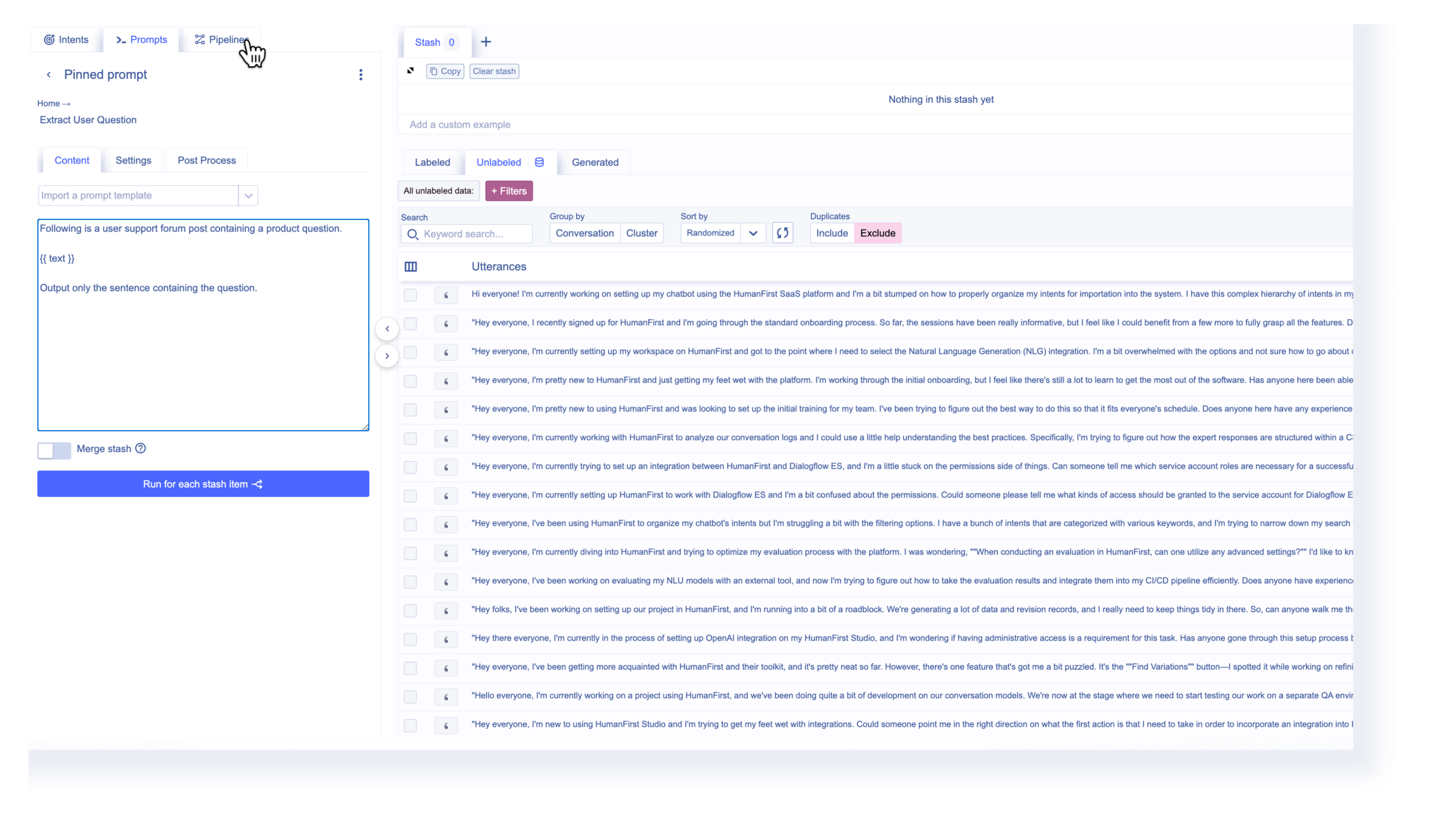
Working with the isolated questions, we used a data engineering feature to rank them by uncertainty. This compares the user questions against the labeled examples. A low uncertainty score means the question would be well covered. A high uncertainty score indicates a gap in the knowledge base content.
.png)
Query-Driven Knowledge Base Completion
We can group the real user questions by topic and quickly see which topics have high uncertainty scores. The demonstration above walks through labeling and tagging those groups.
We now have a new library of missing content; we can view only the content we’ve tagged “missing,” and apply the phrase count feature to see which missing topics had the most user queries. This is the team’s next knowledge article to write, and the group of user questions shows exactly what it needs to cover.
.png)
By comparing user questions against the generated examples of questions the knowledge base could address, the gaps became clear. The query-driven examination was made possible by the apples-to-apples comparison and the process was made infinitely faster by the LLM.
Continuous Knowledge Base Maintenance
Improving RAG tools through knowledge base maintenance requires that teams can understand the knowledge content they have, monitor their user’s questions as they change, and establish a system to continuously fill the gaps. Teams need to be able to save and add to the library of addressable questions and consult the uncertainty scores of user questions as they come in. This workflow creates an open loop for constant customer discovery, low-lift knowledge base maintenance, and RAG system optimization. The risk of hallucination diminishes, and the likelihood of providing customers the most useful, up-to-date comprehensive information compounds.