
Generative AI (gen AI) is on the radar of every enterprise leader, but the extend and nature of it's use is highly varied. While the complex applications of AI have been reserved for machine learning engineers and data scientists, gen AI has introduced non-technical experimentation, and early experiments have employees excited.
.png)
Gen AI extends to almost all areas of knowledge work, which opens the door to non-technical AI adoption. This exciting expansion is a complex initiative that enterprise technology leaders are just beginning to tackle.
In this article, we look at how employees are experimenting with gen AI, the different states of enterprise adoption, and the shifts in thinking, tooling, and training required for workforce-wide AI.
Employee Use of Gen AI
In March of 2023, experts forecast that 80% of the US workforce could augment at least 10% of their tasks with gen AI. By August of 2023, 79% of employees had at least some exposure to gen AI, and 22% were using it regularly.
This August, McKinsey reported 91% of surveyed employees are using gen AI tools in their work.
What Kind of Gen AI?
Non-technical AI adoption is coming primarily from what McKinsey calls “embedded” AI; platforms like OpenAI’s ChatGPT, Microsoft’s Copilot, and Anthropic’s Claude. These and other point solutions, like Jasper for marketing, Apollo.io for sales outreach, and Perplexity AI for writing and research, comprise the bulk of employee-driven AI experimentation.
What Are Employees Using Gen AI For?
If we study the cited benefits among ‘power users’ of embedded AI–employees who use gen AI proficiently and frequently–three categories emerge.
Efficiency (Doing Things Faster). Employees can access vast knowledge that was previously limited to experts, enabling them to make more informed decisions faster. 92% of power users report a more manageable workload.
Automation (Doing More Things). Employees use gen AI to take repetitive tasks off their plate, and take on more work independently. An increased ability to focus on and accelerate their most important work is reported by 93% of power users.
Optimization (Doing Things Better). The available AI tools are also elevating employee’s work. They can consider more sources in the same amount of time; day-to-day decisions can factor in more data. 91% of power users experience more motivation and enjoyment.
.png)
What’s Preventing Broader AI Adoption?
Many companies have endorsed the use of gen AI by covering the cost of those embedded AI platforms (like ChatGPT). But some are understandably skeptical of how much these tools will change the way their employees work, and what risks come with workforce-wide use.
Problem: AI Productivity Gains are Capped
Many of the embedded AI solutions are understood as AI ‘assistants’; they help employees to complete their tasks marginally faster, but don’t fundamentally augment how those tasks are managed. Equipped with ChatGPT, a business analyst might be able to summarize a call transcript faster. But the ensuing tasks — inputting new transcripts, drawing insights across a high volume of summaries, and implementing strategy changes — are no less tedious with a conversation-style AI interface.
In response, vertical solutions have risen to the call, providing some AI-led assistance to many disparate aspects of an employee’s responsibilities.
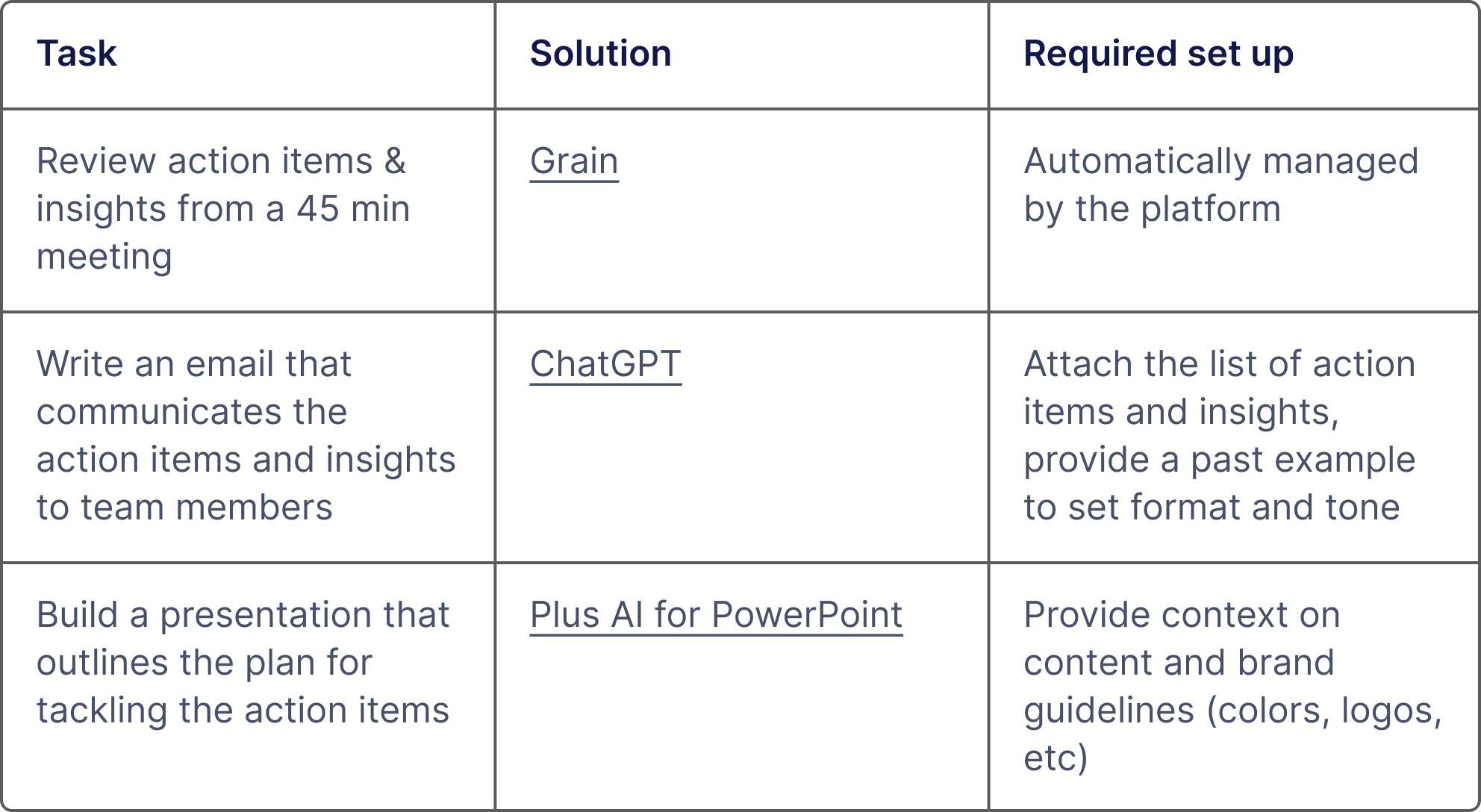
Purchasing so many point solutions can be costly to enterprise organizations (the best AI-enabled tier for Salesforce costing 3x more than the Enterprise tier) and sprawling tool requests force CIOs to decide which solutions to greenlight across which departments.
Investments in point solutions are also inherently siloed; each vertical platform pulls from a different data source (i.e. sales calls, CRMs, customer support environments) and prohibits to any meaningful cross-functional implementation. As productivity increases marginally, software expenses and departmental silos quickly compound.
Problem: Custom Built Solutions are Slow & Incomplete
Company leaders who believe that AI can have a meaningful impact beyond those siloed solutions often invest in building their own. In-house builds require AI-specific talent who can use open-source technology, like Mistral, to train models on company-relevant data and build an interface that’s accessible to multiple teams.

While these projects use data from multiple domains, development timelines can span several months. Constant maintenance is required to improve the models and keep them up-to-date with the current business environment. Without an easy way to pass off the work to business teams, the ROI is capped.
How to Drive Meaningful, Cross-Team AI Adoption
For technology leaders that feel underwhelmed by the existing solutions, and dissatisfied with the adoption of build-it-yourself solutions, there’s a better path toward broader AI enablement. This path involves:
- A better interface through which employees can interact with gen AI.
- Future-proofing the organization’s gen AI strategy with access to multiple models.
- Investing in the education that non-technical AI adoption requires.
A Better AI Interface
Restricting AI to the conversation-style interfaces of ChatGPT and other model providers limits the work employees will be able to do, and keeps the real benefits of gen AI restricted to technical stakeholders. It’s important to view the interface through which teams interact with AI and the model they interact with as different products.
A better AI interface will give users a more controlled, creative, and custom interaction with gen AI models, including the following critical components:
- Data. Even for non-technical teams, real work requires real data. Today, most gen AI tools don't leveragebusiness data, and the ones that do operate with black box processes - employees can't see how they're getting to an end output. In an ideal environment, employees can curate the data that matters to the task, focus on a specific segment, and transform that data at scale using AI, without losing any visibility.
- Prompts. As employees gain comfort and confidence with gen AI, prompts will become increasingly task-specific, custom, and complex. An ideal interface provides employees the space to do the iterative work required to train the AI to manage increasingly complex initiatives and align with their expectations. Instead of being limited to one-off tasks, a powerful prompt engineering workspace allows users to build their AI workflows over time and unlock new high-value projects. Once developed, it’s important that these prompts are easily shared between colleagues and across departments to standardize performance and pick up speed.
- Domain Context. Human expertise is what’s missing from most AI experimentation today. With a user-friendly and flexible interface, domain experts will begin to learn the critical skills necessary to transfer their implicit knowledge and standards to the AI. By engineering prompts against real data, non-technical experts can tailor their AI workflows to their needs and expectations. It’s one thing to use AI to analyze a high volume of data, it’s another thing to train an AI to analyze a dataset the way an expert would. This is where companies will gain the extra mile, which will be the primary source of competitive returns.
With the right interface, employees can build an iterative relationship with gen AI that rewires how they work and encourages lasting, impactful adoption that drastically extends their impact.
Using Multiple AI Models
The enterprise AI model landscape is quickly expanding without an end in sight. Leaders who opt to buy the enterprise tier of a specific model provider (OpenAI, Anthropic) limit their ability to react as the landscape changes. This leads to higher costs and limited usability.
Companies will quickly need to leverage multiple models, including ones that are built and fine-tuned in-house, to empower every team to work optimally with AI. By up-skilling employees on an interface that’s interchangeable across models, organizations can ensure that data and prompt workflows are easily transferable to the model that’s right for the task. This also makes in-house development projects and fine-tuned models accessible to non-technical teams, so the returns of the development projects can scale across the company.
Choosing an interface that connects company data sources and multiple LLM vendors, and that provides employees a means of verifying the output they’re getting, allows business experts to do work that was previously impossibly technical without relying on data and engineering teams. For enterprise technology leaders, that alleviates one of the biggest cost factors related to AI experimentation: technical talent.
Educating Employees on AI
Even the best AI interface is obsolete if employees aren’t well supported in their adoption journeys. For enterprise leaders, education is becoming a key challenge–the task is to empower employees to augment their workload, and to do more of their best work, without adding work to their plate or creating friction wit their existing processes.
With a collaborative interface, the learning journey becomes significantly easier. CIOs can develop training that has the same core concepts, but adapts to the work of individual departments. In a shared environment, employees learn from each other, and progress comes faster than it would on its own.
The following is an example of how educating a workforce on the underlying technology and having them use the same, user-friendly interface, can lead to compounded gains:
.png)
The above example can be done without any input from technical teams, keeping the experts who are closest to the business function in charge of the solution. Not only does this lessen the burden on the technical specialists, it also empowers domain leaders to expand their use of data, and get closer to ground truth insights in every aspect of their work.